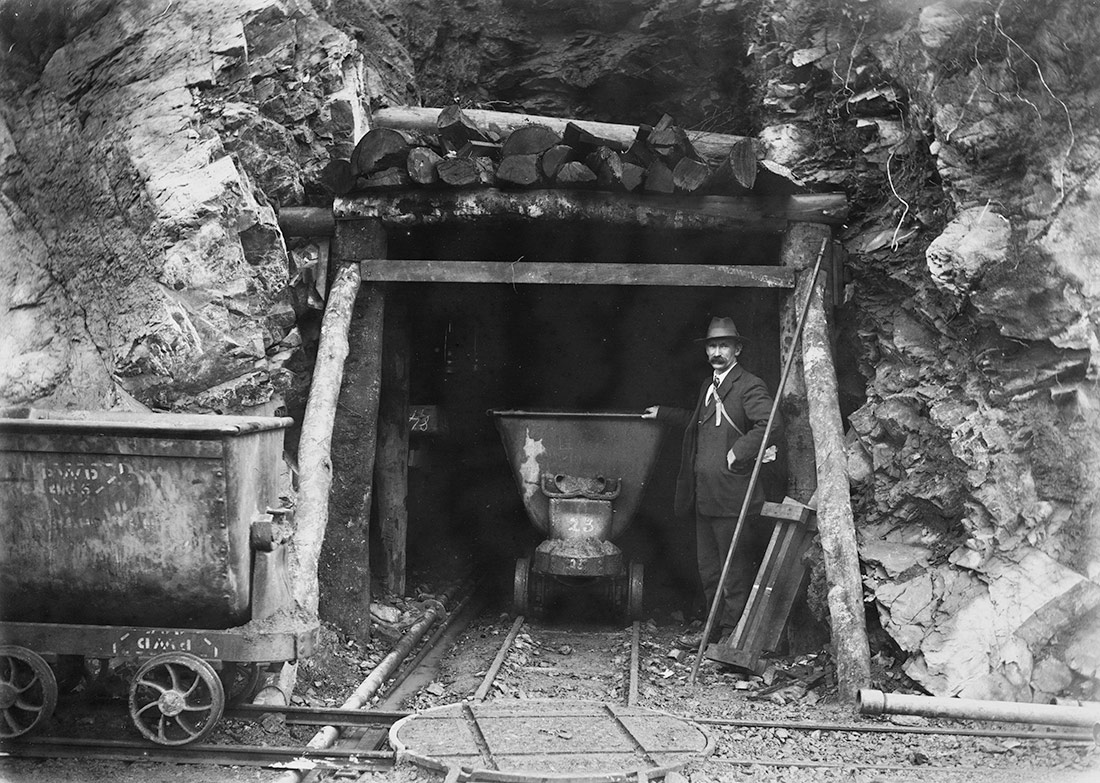
Entrance tunnel at the Mangahao Power Station. Shannon, New Zealand, 1921 | Leslie Adkin, Te Papa | No known copyright restrictions
While we believe in the idea of an AI as an abundant resource with no physical existence, we are also confronted with the proof and consequences of its materiality. We wonder, however, to what extent we can understand the complex web of water, minerals, energy sources, data and labour that make up these systems. As well as their environmental impacts and our responsibility for them.
> Are you thirsty, ChatGPT?
My finger pauses over the enter key. Researching the material infrastructure and environmental impact of computation has attuned me to the absurdity of putting these enormous, energy-intensive, and almost entirely invisible technical systems to such apparently frivolous use, and the paper I’ve just read – “Making AI less ‘Thirsty’” by Li Pengfei and colleagues, which suggests that a 20-50 question interaction with ChatGPT will consume 500 ml of potable water, and which had prompted the question I just typed – heightens this ambivalence in my mind. My habits are somewhat behind my conscious doubts though, as the long history of previous chats in the ChatGPT sidebar attests. It’s almost too easy to query it about whatever comes to mind. I press the key.
“As an AI, I don’t have feelings, physical sensations, or needs, so I don’t experience thirst or any other human conditions. But I’m here to help with any questions or tasks you have in mind!”
This idea that ChatGPT is an abundant, immaterial resource, without any physical existence, but always available for whatever query might occur to me, already ingrained in its affordances and my nascent habits of use, is confirmed by its response. I press further:
> What are you made of, ChatGPT?
“I’m made of code and data! Essentially, I’m a complex algorithm that processes and generates text based on patterns and information learned from a huge amount of text available on the internet. My ‘brain’ is made up of various machine learning techniques and neural networks that help me understand language and provide responses.”
> Where are you, ChatGPT?
“I exist in the digital realm, residing on servers and computers where I can access information and interact with users like you. But in a more abstract sense, you could say I’m wherever you access me from – a website, an app, or any platform that hosts me!”
At every step, I find that ChatGPT insists on its own immateriality, while at the same time, I’ve just been reading empirical work which lays out in stark terms the many and varied material supports (and material consequences) of ChatGPT as a technology. I find this significant for two reasons.
The first is that I suspect this is not entirely accidental. Lucy Suchman, an anthropologist of technology and one of the founders of the discipline of human-computer interaction, in her recent article “The Uncontroversial ‘Thing-ness’ of AI” identifies how the fact that the term ‘AI’ functions as a ‘floating signifier’ reinforces the suggestive power we imbue it with: the supposed immateriality of ‘AI’ is a contributing factor in the authority we imagine in its knowledge.
The second is that in comparing ChatGPT’s own utterances to the investigation of Li and colleagues, and many others probing the material footprint of AI, we’ve encountered a controversy – on one hand, an insistence that AIs like ChatGPT have no material existence, and on the other, the assertion that their material existence and its consequences are both considerable, and of considerable importance. Controversies, as the work of STS scholar Tommaso Venturini shows, offer us opportunities to learn – they’re “the best available occasions to observe the social world and its making.” The presence of a controversy, then, offers us an opportunity to better understand the hidden socio-material aspects of this supposed thing, ChatGPT, which presents (and describes) itself as being disembodied, ethereal, even while we’re confronted by the evidence and consequences of its very material existence.
Taken together, these two opportunities point to a two-fold purpose of this sort of research: the way in which the materiality of an AI system is entangled with its epistemic and epistemological qualities (both what and how the machine comes to ‘know’), and the ways in which we can come to know it. The specific type of intelligence that AI is, and our beliefs about it, are based on its materiality, which allow us to probe both the limits of our knowledge about it, and of its knowledge of the world through our investigation of its material infrastructure.
We could call this manner of studying AI an ecological approach, and it is ecological in two distinct senses. First, we’re interested in the ecology of AI in terms of its material impact on the environment, but we’re also interested in AI as an ecology in itself: as Matthew Fuller describes it, a “massive and dynamic interrelation of processes and objects, beings and things, patterns and matter” which includes both its material, epistemic and phenomenological aspects, rather than as a discrete thing, material or otherwise.
So, what things are included in this material ecology of ChatGPT? This is what I and the participants in the “Minds, Lies and Materials” workshop attempted to uncover, one Saturday afternoon in January as we mapped out the multiple material bases of ChatGPT and the controversies that revolve around them. Investigating and contrasting multiple perspectives (our own investigation using technical tools and Open-Source Investigation methods, along with published independent research, official announcements by OpenAI and the utterances of ChatGPT itself), we focused on the sources of energy, water, data, labour and minerals which make up the supposedly disembodied ‘intelligence’ of ChatGPT. This allowed us to map their sources, relationships and movements in a manner inspired by Cartografias da Internet and Kate Crawford’s Atlas of AI, and aided throughout the whole process by Anne Pasek’s methods for Getting into Fights with Data Centers.
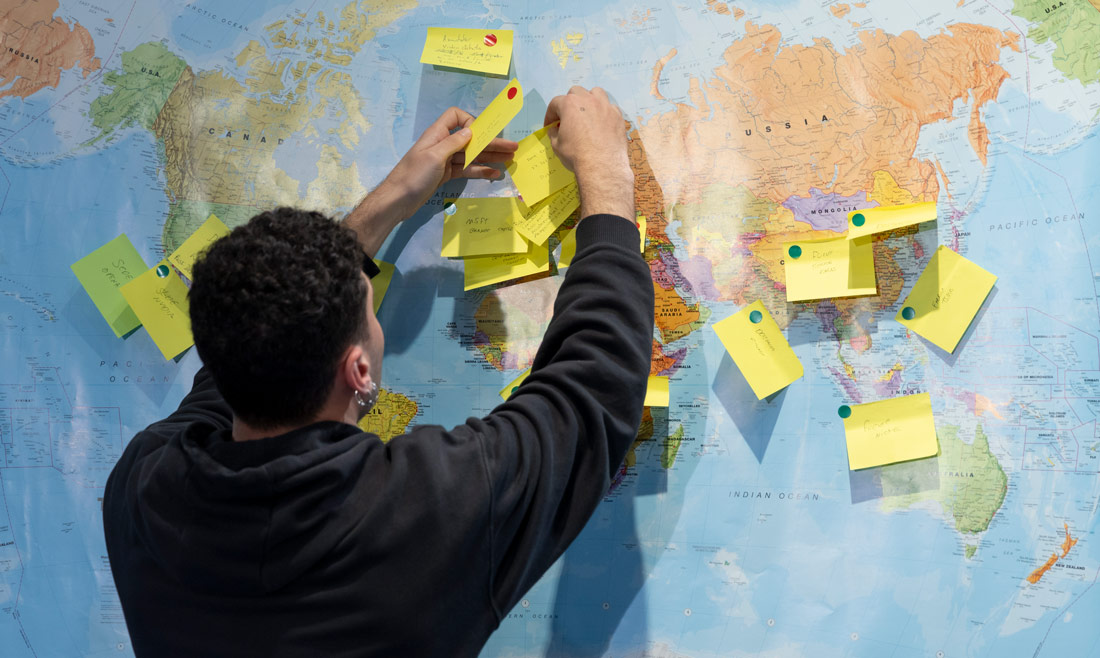
Workshop: Minds, Lies and Materials. CCCB, 2024 | CC BY-NC-SA Pau Fabregat
This was a provisional, speculative exercise – we did not expect to settle these controversies one way or another, but rather to attune ourselves through an open-ended process of investigation to the many material facets of AI, employing something akin to the Rubber-Boots Methods of curiosity, collaboration and critical description (even though on this occasion our feet didn’t leave the carpeted floors of the CCCB).
We ended up with a map, or rather three overlaid maps. The first resulted from our own research, and showed a dense network of water from a reservoir serving a populous Dublin suburb (the excessive use of which by data centres has already raised controversy), the labour of underpaid data annotators in Kenya, and the Irish electricity grid – which boasts two local hydroelectric stations, but which Microsoft plans to augment with a dedicated gas-fired plant. It also included data gleaned from the web, nominally globally, but in fact with a notable bias towards the English-speaking parts of the global north. The rest of the globe contributed mainly in a more tangible form, with Nickel from Indonesia, Cobalt from the Democratic Republic of the Congo and Lithium from Chile being brought together in GPU factories in Taiwan and ending up as e-waste in China, India and Nigeria. Overlaid on this, a second map: OpenAI’s presentation of itself as a headquarters filled with ingenious scientists and innovators in San Francisco, along with a data centre filled with high-tech servers in Des Moines, Iowa. The third ‘map’ hovered uneasily outside the frame: ChatGPTs insistent description of itself as disembodied and ethereal.
This method was not without its problems. In attempting to open the black box of ChatGPT, we encountered still more black boxes inside – parts of the system which were opaque and unknowable from our perspective and with the tools and methods at hand. However, having chosen to contrast our own perspective with that of ChatGPT itself, and that revealed publicly by OpenAI, these frontiers of knowability became results in their own right. Identifying the differences between the types and depth of knowledge available to us from each of the different sources we identified made us newly sensitive to the limits of each.
This also involved the realisation that we were not just studying this controversy, but were ourselves a party to it, through our investigation from a situated, partially-informed perspective, leading us to confront situations in which our own first-hand experience contrasted with or contradicted the claims of another source, which on the surface could claim more authority.
A case in point – using traceroute and some other slightly-hidden technical information on the ChatGPT site that suggested we were communicating with a server in Europe, along with a few inferences gleaned from publicly-available information (that Open AI uses infrastructure owned by Microsoft, whose currently-operational European data centres are in Dublin and Amsterdam), we settled on Microsoft Grange Castle, Dublin, as a likely location and focus of further study, only later to discover a footnote in some OpenAI technical documentation which stated that their infrastructure is located in the US (likely Iowa). More than just a mistake however, this proved to be generative of some useful discussion. Should we place more faith in situated and partial first-hand methods, or in opaque claims made by the business itself, when trying to understand an object of study (ChatGPT in its material form) which we can’t directly access? For our immediate purposes this may not matter so much: digital infrastructures such as ChatGPT are built to scale, i.e. they’re designed to expand uniformly, being composed of smaller, modular and identical units, analogous to Lego bricks or shipping containers. One consequence of this uniformity is that it makes the knowledge gained in one place more easily transportable – what we learn from looking at Dublin can still teach us about a data centre in Des Moines, because they’re designed for uniformity. In this way, our knowledge of scalable systems can perhaps be turned into a technique for ‘resisting scale thinking’ – using the similarities between infrastructures in different places as a tool for comparing, contrasting and building solidarities between them.
At the end of the workshop, reflecting on the complex web of minerals, water, energy, data and labour which we discovered, as well as the blind spots, ambiguities and contradictions we encountered, we debated how this research might inform our attitudes to and interactions with ChatGPT and other AI technologies in the future. Having been made aware of the limits of our own ability to know the material effects of these systems, as well as the oblique, selective, and sometimes even dishonest way in which both OpenAI and ChatGPT present them, two major doubts emerged: what can we do to mitigate the environmental consequences, and is it our place, or our responsibility to do so?
One possible answer to these questions arose through the discussion of a third doubt: was this massive, wasteful and centralised model of computation strictly necessary? We recalled earlier attempts at distributed super-computation, such as SETI@Home, which recruited idle home computers around the world to solve complicated scientific tasks, and asked: is the massively centralised, wasteful architecture of ChatGPT really necessary for artificial intelligence? We came to the conclusion that perhaps, in some narrow sense, it is – Generative AI models such as ChatGPT arose from particular innovations in computer hardware (the development of the GPU) and the particular economic and technological conditions of surveillance capitalism which allow the appropriation of data on a large enough scale to train these models, both of which, in some sense, imply this centralisation as a necessary condition. However, through our awareness of these material conditions, we came to realise that perhaps what is needed is an amplification, or reframing of our idea of AI itself. To follow Yuk Hui’s call for a renewed attention to technodiversity – and, through imagining other, less extractive, material configurations for AI, collectively conceive of other, more liberatory and convivial ways in which machines might be considered intelligent.
Leave a comment