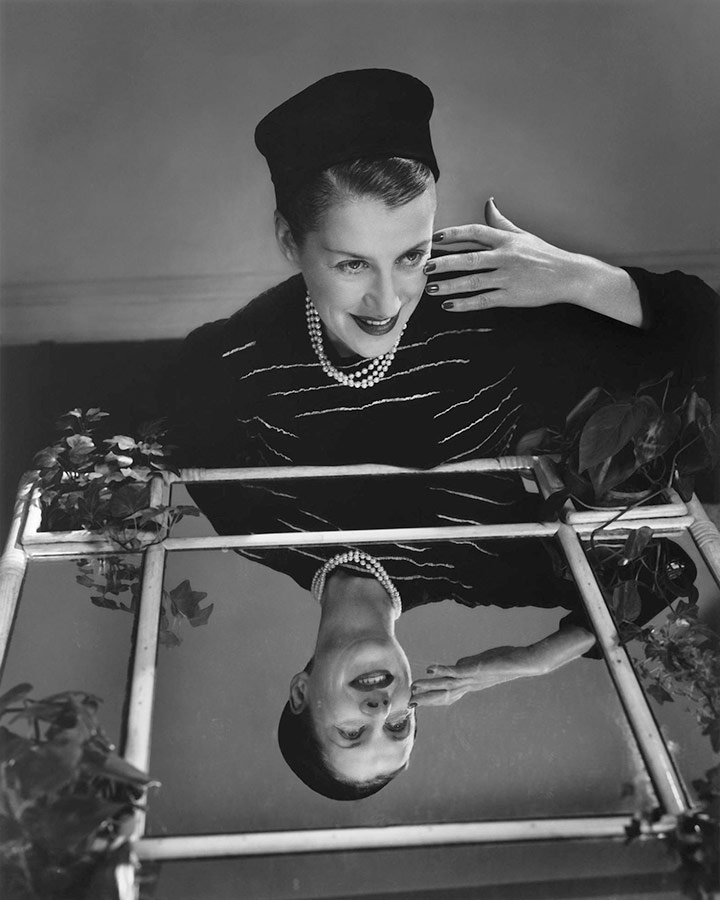
Beatrice Lillie, New York 1948 | Yousuf Karsh, Library and Archives Canada | Public Domain
Generative AI is a matter of statistics, an imitation of the style of the dataset used to train it. Questioning how this process plays out leads us to reflect on the tool’s abilities and limitations, as well as the value of its results. These are the reflections offered by the collective Estampa through two installations that experiment with the CCCB Archive using AI.
The field of artificial intelligence has made progressive inroads into different areas. One of the latest spheres into which it has expanded is generation, with services that offer seemingly automated content creation. These generative AI tools work through imitation. They are designed so that, when given a set of data (text, image or audio), they will look for optimal statistical solutions of pixels, letters or sounds which could also fit into the dataset. In other words, they try to reproduce a style through an act of camouflage. A good expression to use would be “piecing together.”
Like any disguise, implicit to these tools is a process of analysis – what are the most common characteristics of that which they are attempting to mimic? What motifs are repeated? How are the elements combined? Generative AI is a mirror, more or less realistic, more or less distorted, of what we ask it to imitate; an analysis of the dataset used to train it.
Nowadays, any archive is not only a place for conservation, research and dissemination, it is also a possible dataset. One of those sets of data from which the style can be extracted. This is how, for the installation The Infinite Talk, the CCCB’s archive of talks has become the source material for a text generation network. The network pieces together the talk genre and the type of speech used; it combines the words to create conversations that might possibly have taken place here – texts that could also pass for material from the archive. Imitation opens the door to analysis, to a reflective gaze in the mirror, and also, at times, to the surprise of unexpected combinations, some plausible, some incongruous.
Although AI is often spoken of as a way to reproduce or imitate human characteristics (an imitation that, in our view, should be used for reflexive analysis rather than camouflage), it is also important to understand what it is about AI that is clearly different. A key aspect of this difference is scale. The writing produced by the network is infinite and rapid; it could go on forever, it can always be asked to generate something else almost instantaneously. In this sense, it is analytical not in an assertive way, but in a propositional way – there is always another possibility, a new variation, a different but similar change of clothes… There is no solution (no conclusion of what has been read; no supposedly ideal text), but an apparent stream of possible forms, a babble that flows when we open the tap.
A second relevant aspect is that AI tools are automatic, and they reflect two synonyms of this word: they are compulsive and reflexive. A network can only do what it does, and it can only do it compulsively – it cannot become silent, for example, or stop mimicking the dataset on which it has been trained. In this sense, AI could be understood as the construction of social reflex actions, as a large-scale process automation based on imitating specific prior decisions. Like a social muscle that contracts according to how individual muscles have previously flexed and unflexed, AI is a reification of what has happened. When it is described to us as the future, perhaps it would not hurt to be aware of the extent to which it is the past made solid.
As we have already mentioned, AI is like turning on a tap, but this can also be said in another sense – just like the management of running water, AI is also an infrastructure. It is not inside our devices, rather it relies on computation performed on distant servers with industrial hardware, while data travels back and forth. It is also an infrastructure because it requires training that can only be done with large computational tools, in very high-capacity and high-consumption data centres. This is the nature of industrial AI, of the generative tools with which we are all familiar and that have been trained with very broad datasets – an extensive technique that widens the number of styles that can be imitated (known in the field of text generation as large language models). All this aggravates the problem of the materiality and energy consumption of the digital world (it is estimated that training ChatGPT consumed as much as the annual electricity consumption of one thousand US homes, and that its current use consumes the equivalent of 33 thousand homes; a figure that is set to multiply rapidly, considering the rate at which these technologies are being implemented in all kinds of tasks). This conception of AI is also underpinned by the dubious extractive logic of the commercial internet, which regards everything that happens and all that we do on the web as material to be used and monetised.
Given this fact of AI as an infrastructure, the generative network used for The Infinite Talk has been trained at the local level, with a high-performance user-level computer, and focusing on a specific dataset: the transcription of the talks given at the CCCB and kept in the archive.[1] Choosing not to use corporate services has made it possible to avoid their larger carbon footprint, as well as the generic and often clichéd nature of the results produced by these industrial generative AI tools. And when comparing the two, although this network would lose in linguistic and textual coherence compared to the latest version of GPT, it would perhaps win in terms of unusual ideas or unexpected outputs.
At the heart of this project is a series of questions about AI and its powers and limitations. Who is speaking in the texts that are generated? What value do we place on the outputs? How do we want to understand them? What is the relationship of statistical variation, and its potential scale, with veracity? What does AI do with what it gobbles up?
Apart from the ecological and stylistic considerations mentioned above, working with a small model allows us to ask these questions on a relatively manageable scale. When presented with the network’s output, we are more likely to question what it is that it does and how far it is able to go. We see not just a result, but the statistical interplay that underpins it, and thus question the tensions between what are statistically considered to be plausible variations and what we ourselves consider these to be. The seams of imitation become visible without unravelling the outfit. We think it is important to keep this perspective in mind, and to apply it to large models and to all the possible uses of AI. Every result of an AI tool must question the network that generated it.
[1] The network used was given an initial generic training with a broad dataset, which functioned as a kind of language learning. We did a second training (also called model refinement) with just the transcriptions from the CCCB archive. Although this initial training was generic and predetermined, it was on a different scale to the industrial models. In the case of the Question Time installation, pre-trained networks are used. One is used to convert the talks archive into a questions-and-answers dataset (the instruction given to the network is to summarise each paragraph as a question) and another is used to write the final answer, based on text fragments from the archive. The training of these networks is out of our control, but both have been used locally on the project computer to avoiding working online.
Leave a comment