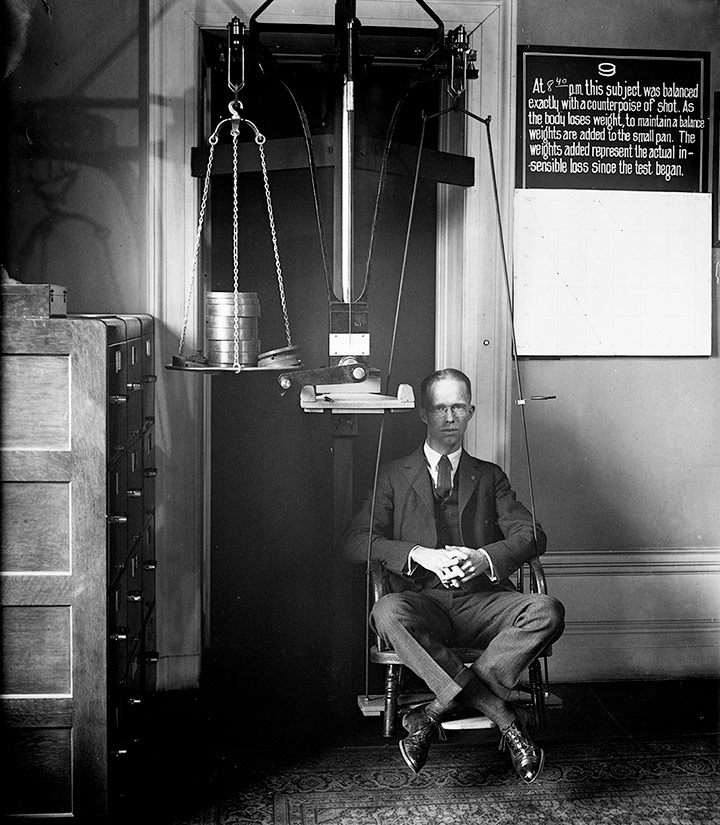
Un hombre en una balanza, 1923 | Harris & Ewing, Library of Congress | Sin restricciones conocidas de derechos de autor
Whether due to the way they have been designed or the way they have been trained, many algorithms have biases. But we humans, too, have lots of prejudices that are often very difficult to identify and also affect our decisions. Detecting in which fields the inclusion of automated systems is most possible and creating fair, transparent algorithms is crucial to generating more just solutions.
In August 2020, secondary school students in Britain took to the streets to demonstrate against the automated system that the UK’s Office of Qualifications and Examinations Regulation (Ofqual) had applied in correcting their exams. As the students had missed classes, the teachers were asked to provide an estimated grade for each student, and a comparative ranking with class mates in their school. Ofqual’s algorithm also took into account the school’s performance in each subject over the previous three years. The idea was that the results should follow a similar pattern and that 2020 students not be at a disadvantage. But the algorithm ended up reducing the teachers’ evaluations in 40% of cases because the calculation gave less importance to each student’s history and teachers’ assessments and more to other, external factors, such as the quality of the school or its past success. This led to visible discrimination against ethnic minorities from poorer regions. This is how brilliant students from schools with fewer resources saw their marks downgraded, with the direct consequence of not being able to go on to university.
Artificial intelligence is effective in predicting major patterns and relationships from big data, as well as streamlining processes. To do so, algorithms are used in ways which are not always neutral. Either because the data with which they are trained has biases, or because biases have been added during their design. As David Casacuberta, lecturer in Philosophy of Science at the UAB, reminds us, the problem is that automated systems, if fed with biased examples, will end up reproducing and even enhancing those same biases. “If, in a country such as the United States, people with African ancestry have much higher possibilities of ending up in prison without bail, and we train a neuronal network with those data, the algorithm ends up replicating the same types of biases.” Proof of this is the research that the independent foundation Pro Publica led for years, until it showed that the Correctional Offender Management Profiling for Alternative Sanctions (COMPAS) algorithm always gave a higher probability of committing crimes for black and Hispanic than white defendants.
If it is known that algorithms can have biases, and that these can cause harm to individuals or groups, why are they used? One answer is that the benefit or accuracy of the results is greater than the damage or error. Is this fair to those who lose out, like students with downgraded marks?
Not all bias is negative
When we talk about bias, we always think of it negatively, but it can also be positive. And this in itself is a language bias. This occurs when we seek gender parity or want to take an affirmative action to mitigate discrimination against a group of people (positive discrimination). For example, when a company with more than 50 employees is required in Spain to have at least 2% of employees with a disability.
An intrinsic problem with bias is that we often know in which direction we need to go to correct it, but not how far. For example, it is not clear that there should be gender balance in all professions. Nursing is one example of a profession where women have shown greater empathy with patients, which would justify their being in the majority. The right distribution may not even exist, or it may have to be based on social consensus. In the case of affirmative actions, the perception of positive bias may be more important than the action itself. Suppose an engineering faculty wants to increase the proportion of female students, currently just 20%. To do so, it decides to grant 5% of its places (the last ones) just to women, which would serve to increase the proportion of women annually by 1%.
This example is not hypothetical, but has actually occurred at the University of Chile since 2014. Currently, this quota has increased to 8%. An analysis of this program has shown that the difference between choosing women or men is one more right answer in the maths exam, which is not statistically significant and, therefore, there is no real gender discrimination. What is more, in five years the percentage has exceeded 30%, since the perception that it is easier to enter and that there will be more women has increased the habitual number of female applicants. That is, perception of the action was more powerful than the action itself (an increase of 7% as opposed to the 5% represented by the action). Recently, an exclusive 1% has also been added for indigenous Mapuche inhabitants. This success has now been replicated in almost all engineering schools in Chile.
Fairness and algorithmic transparency
Sometimes, bias leads to a claim for algorithmic transparency by citizens, when the AI system has been seen to be unfair. To understand how an algorithm made the prediction or decision, we use the term explicability (explainable artificial intelligence). Transparency is an ethical principle recognised by the European Union in numerous documents such as the Ethics guidelines for trustworthy AI. The main objective is to prevent discrimination by the automatisms of algorithms largely due to biases contained in the data with which AI systems are trained.
An example of this kind of failure would be the Bono Social, an economic compensation that the Spanish government promised in 2018 but that has brought controversy. It was initially intended to help the neediest families with their electricity bill. To do so, electricity companies checked that applicants met the requirements using a computer application (BOSCO) that decided who should receive these grants. Faced with numerous complaints from families that objectively met the requirements but had their application turned down, the citizen foundation Civio asked for information about the design of the algorithm and the source code of the tool, in accordance with to the Transparency Act. “If we do not know the inner workings of applications like this, we cannot supervise the work of the public authorities”, said David Cabo, director of Civio. The idea was to identify where in the process there could be errors and, having detected them, do everything possible to ensure that families received their social compensation. Faced with the government’s refusal, they have filed a contentious-administrative appeal.
A researcher at the Oxford Institute, Sandra Wachter, thinks we should have the legal right to know why algorithms make decisions that affect us. She explains that governments, companies and financial organizations should give “counterfactual explanations”—that is, if you’ve been denied a mortgage, you should be able to ask the bank: “If I earned 10,000 euros more a year, would you have granted me the loan?” But for some experts, such as Kate Vredenburgh of Stanford, believe that this type of explanations also can have bias.
Ethical principles to avoid bias
To summarize, we could list some ethical principles associated with having less bias in AI-based systems. There are dozens, but the most important ones related to bias are:
- The algorithm must be fully aware of what it is doing. In this case, it needs to be aware of its biases and how to mitigate them.
- The algorithm must be fair and not discriminate against people. One of the most important causes of inequity is negative bias. An alternative that reflects fairness is for the algorithm to be interpretable and/or explainable, and this means addressing existing biases.
- The algorithm must be transparent—that is, fully reflect how it works, including if there is bias in the data, if there is bias added by the algorithm or if there is bias produced in the user’s interaction with the system. If there is not enough transparency, the algorithm must be auditable to be able to verify, for example, that someone has not been discriminated against. Note that transparency is required by lack of confidence, since in the Anglo-Germanic world people trust the system, and accountability is ultimately required.
As yet unresolved…
Finally, let’s go back to our question. If we use algorithms knowing that they have bias, why do we let them make decisions? One answer is that humans also make mistakes in our decisions, largely due to the prejudices we have acquired. Biases—particularly cognitive biases—are like prejudices: very difficult to identify. The person who has most prejudices is the one who thinks they have none. But we can also look at it from another viewpoint: automated systems are of great help in situations where bias does not play an important role, such as air traffic control. Having professionals working long hours under tension is more dangerous than training machines for the task. They don’t get tired; they are programmed and they are more efficient.
Another answer is that algorithms are fairer than people because, given the same data, they always make the same decision. People, conversely, have much more “noise”, making their decisions more random. This is a major problem in court, where judges’ mood may be even more influential than their biases. Indeed, along with other authors, Daniel Kahneman, Nobel Memorial Prize in Economic Sciences, alerts us to the high cost of inconsistent decision-making in humans and the fact that algorithms are, in general, fairer.
How can AI help us create a more just world? A partial solution would be to create a Jiminy Cricket-type virtual assistant who could alert us when it detected prejudice, whether in speech, action or judgment. Or warn us in the event of possible manipulation based on bias integrated into an intelligent system. But how many of us would agree to let a machine—a mobile phone or device—listen in permanently to what we say (in private and in public) to correct these prejudices and biases?
ruizdequerol | 17 March 2021
Felicitaciones por un artículo reflexivo y bien documentado.
Sólo una pregunta aviesa: ¿Cómo puede ser un algoritmo “completamente consciente de lo que hace”?
Muy cordialmente
Leave a comment